Artificial intelligence helps to identify correct atomic structures
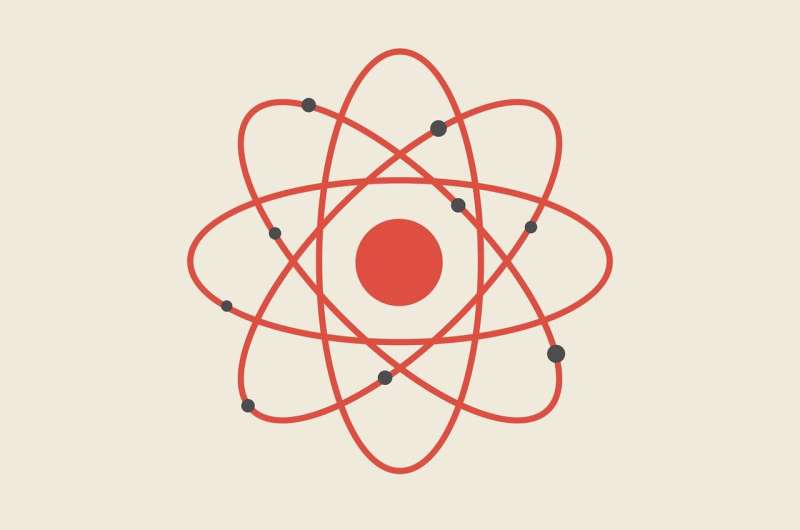
Functional materials are commonly employed in emerging technologies, such as green energy solutions and new electronic devices. These materials are typically blends of different organic and inorganic components and have many advantageous properties for novel applications. To achieve their full potential, we need precise knowledge on their atomic structure. State-of-the-art experimental tools, such as atomic force microscopy (AFM), can be used to investigate organic molecular adsorbates on metallic surfaces.
However, interpreting the actual structure from microscopy images is often difficult. Computational simulations can help to estimate the most probable structures, but with complex materials, accurate structure search is computationally intractable with conventional methods. Recently, the CEST group has developed new tools for automated structure prediction using machine-learning algorithms from computer science.
In this most recent work, researchers have demonstrated the accuracy and efficiency of the Bayesian Optimization Structure Search (BOSS) artificial intelligence method. BOSS identified the adsorbate configurations of a camphor molecule on a Cu(111) surface. This material has been previously studied with AFM, but inferring the structures from those images was inconclusive. Here, the researchers have shown that BOSS can successfully identify not only the most probable structure, but also eight stable adsorbate configurations that camphor can have on Cu(111).
They used these model structures to better interpret the AFM experiments and concluded that the images likely feature camphor chemically bound to the Cu surface via an oxygen atom. Analyzing single molecular adsorbates in this way is only the first step toward studying more complex assemblies of several molecules on surfaces and subsequently the formation of monolayers. The acquired insight on interface structures could help to optimize the functional properties of these materials.
More information: Jari Järvi et al. Detecting stable adsorbates of (1S)-camphor on Cu(111) with Bayesian optimization, Beilstein Journal of Nanotechnology (2020). DOI: 10.3762/bjnano.11.140
Provided by Aalto University